Digital pathology AI and radiomics model development
Work package four
This work package improves digital pathology Artificial Intelligence (AI) interpretation of nodule and cancer histology and develops a radiomics model.
The potential of radiomics has been demonstrated on retrospective cohorts.
Assembling a large prospective cohort of treatment-naive patients from the LHCs dramatically reduces the data bias inherent to retrospective cohorts. The histology slides of those receiving follow-on treatment and associated biopsy samples with highly localised regions identified by super voxel segmentation allow for deep learning to identify novel features. In addition to traditional TNM (Tumour, Node, Metastasis) staging and grade, we are able to evaluate the scope of using features that capture the morphology of relevant histological patterns. This work package builds on our experience of applying domain adversarial learning to large histology data sets to suppress features that could be specific to a particular scanner or training data set.
To learn more about this work, please see the paper on “Contrastive Representations for Continual Learning of Fine-Grained Histology Images” presented by Dr Rohan Chakraborty at the 2021 Machine Learning in Medical Imaging (MLMI) conference.
Our digital pathology AI work package is led by Prof Clare Verrill and Prof Jens Rittscher, supported by Dr Rohan (Tapabrata) Chakraborty, Dr Mengran Fan and George Batchkala. Roche Diagnostics is the industrial partner.
Researchers who work on this package
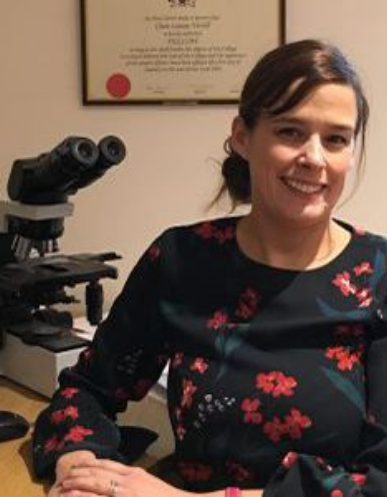
Prof Clare Verrill
Digital Pathology AI and Radiomics Model Development (WP4)
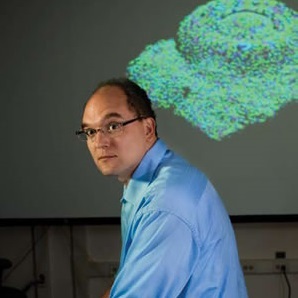
Prof Jens Rittscher
Digital Pathology AI and Radiomics Model Development (WP4)
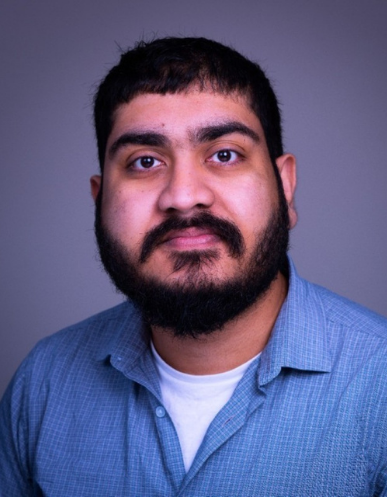
Dr Tapabrata (Rohan) Chakraborty
Post-Doc, Digital Pathology AI and Radiomics Model Development (WP4)
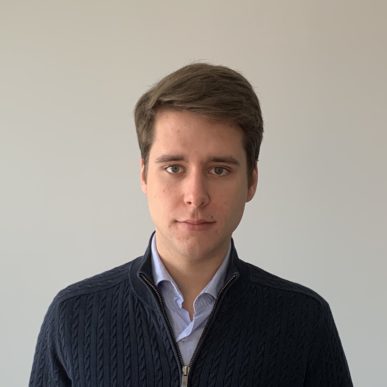
George Batchkala
Digital Pathology AI and Radiomics Model Development
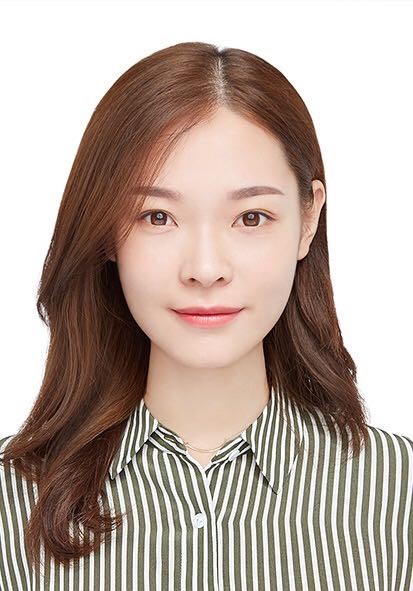
Mengran Fan
Digital pathology AI and radiomics model development