About
DART is using data collected by the NHS Lung Health Check programme to develop and validate Artificial Intelligence to more accurately and quickly diagnose lung disease
Area of work
Lung cancer
Imaging modality
Medical records; CT scans; PET scans; digitised pathology slides; blood samples
Size of data set
145000
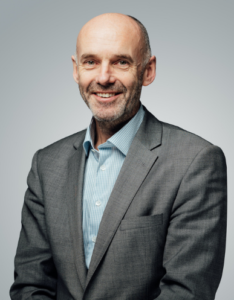
Project lead
Prof Fergus Gleeson
Number of NHS partners
17 NHS Trusts
The DART consortium
A team of academics and NHS clinicians have joined with three leading industry partners to use clinical, imaging and molecular data collected by the NHS England Lung Health Check programme, to define a new set of standards for lung cancer screening. The Lung Health Check (LHC) programme will increase the number of lung cancers diagnosed at an earlier stage, when treatment is more likely to be successful.
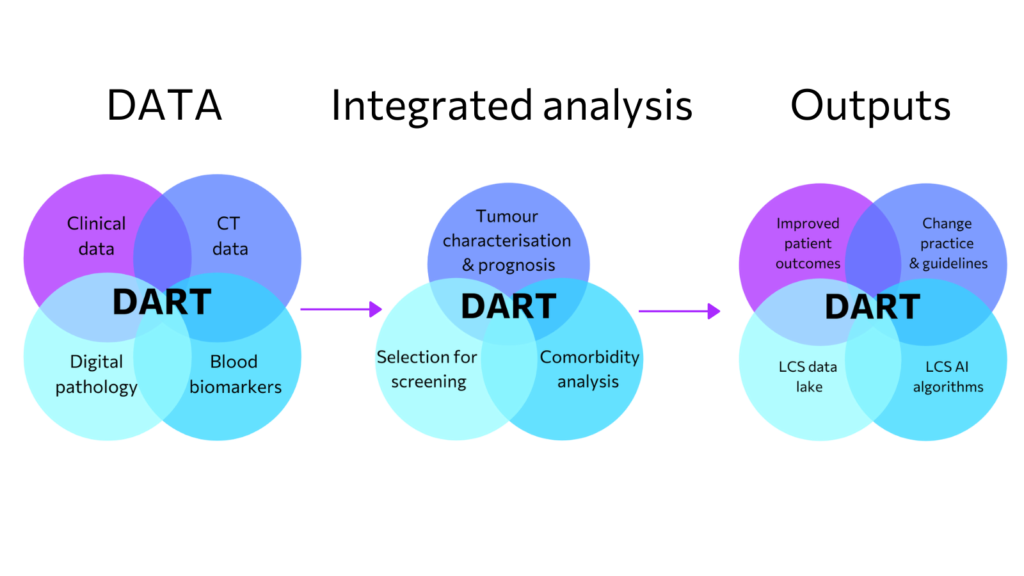
The DART Consortium is collaborating with the Lung Health Check programme to embed research into this national screening activity. Artificial Intelligence algorithms are being developed, validated and used in novel ways to more accurately and quickly diagnose and characterise lung cancer, resulting in fewer invasive clinical procedures.
DART’s full title is “The Integration and Analysis of Data Using Artificial Intelligence to Improve Patient Outcomes with Thoracic Diseases”.
Data received from Lung Health Checks and sent to academic and industrial partners exceeds
- 227,000 clinical records
- CTs for 80,000 patients
- Pathology slides for 280 patients and
- 500 blood samples.
DART Project aims and objectives
Randomised controlled trials show lung screening can reduce mortality by 20-33% and detect co-morbidities. In 2020, NHS England launched a four-year Lung Health Checks programme, at a cost of £70 million. 600,000 people aged 55-74 who are at higher risk of lung cancer are being invited to participate in a lung health check and, if necessary, a low-dose CT scan at 10 sites in England.
To improve patient care beyond the current screening guidelines, DART, working with the 10 pilot Lung Health Check sites (LHCs), is collecting data from the sites and their onward referral hospitals. Clinical, imaging and molecular data will be integrated for the first time using AI algorithms, with the aim of earlier and more accurate diagnosis of lung cancer. DART builds on existing infrastructure from the National Consortium of Intelligent Medical Imaging (NCIMI) and exploits the multidisciplinary strengths of the team.
DART aims to generate disruptive integrated diagnostic innovations that:
- more accurately diagnose lung cancer with enhanced prognostic information
- reduce the occurrence of harmful invasive procedures in the diagnostic pathway
- improve patient selection for lung cancer screening and reduce costs
- improve assessment of risks from comorbidities; such as chronic obstructive pulmonary disease (COPD)
- improve patient outcomes
- generate and store a large amount of data that can be used for future research in a “data lake”
DART’s aim is to transform patient care, ultimately changing guidelines. The clinician investigators are internationally leading researchers in lung cancer, respiratory medicine, cardiothoracic imaging, and respiratory pathology, and have previously collaborated successfully on numerous projects. Institutionally, they represent the Brompton Hospital and Imperial College, Nottingham University, University of Oxford, Royal Marsden, and UCLH. Together, they enable engagement with the wider patient community.
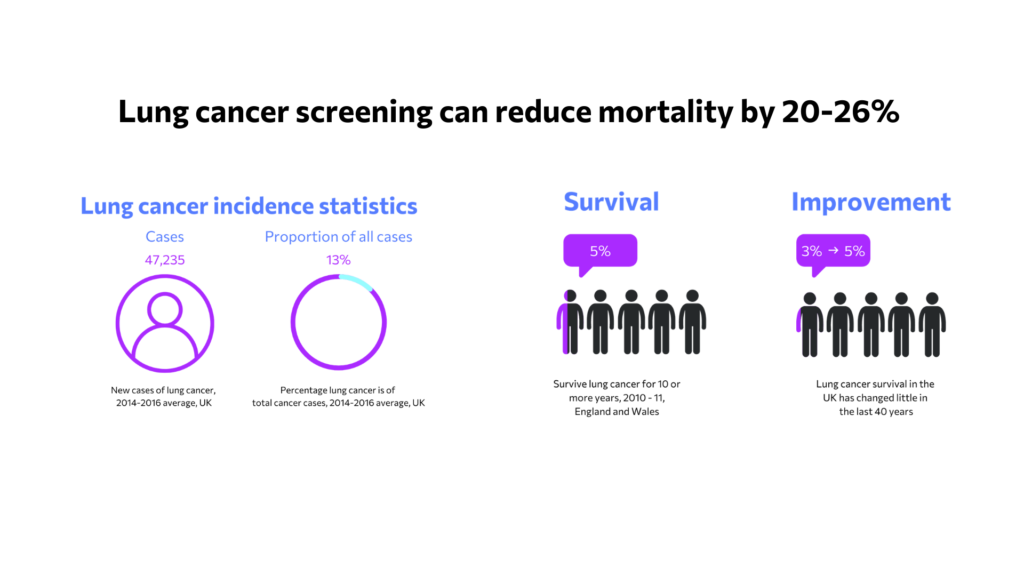
DART is funded by UK Research and Innovation (UKRI) and CRUK, with in-kind contributions from the industry and charity partners.
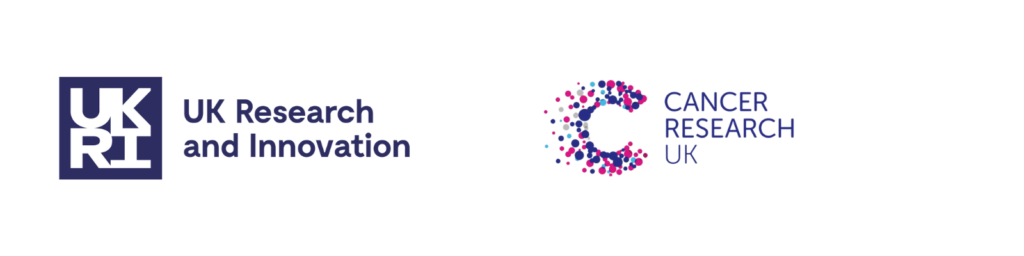
Latest
See all latestActive data enrichment by learning what to annotate in digital pathology
March 11, 2024
Abstract: Our work aims to link pathology with radiology with the goal to improve the early detection of lung cancer.…
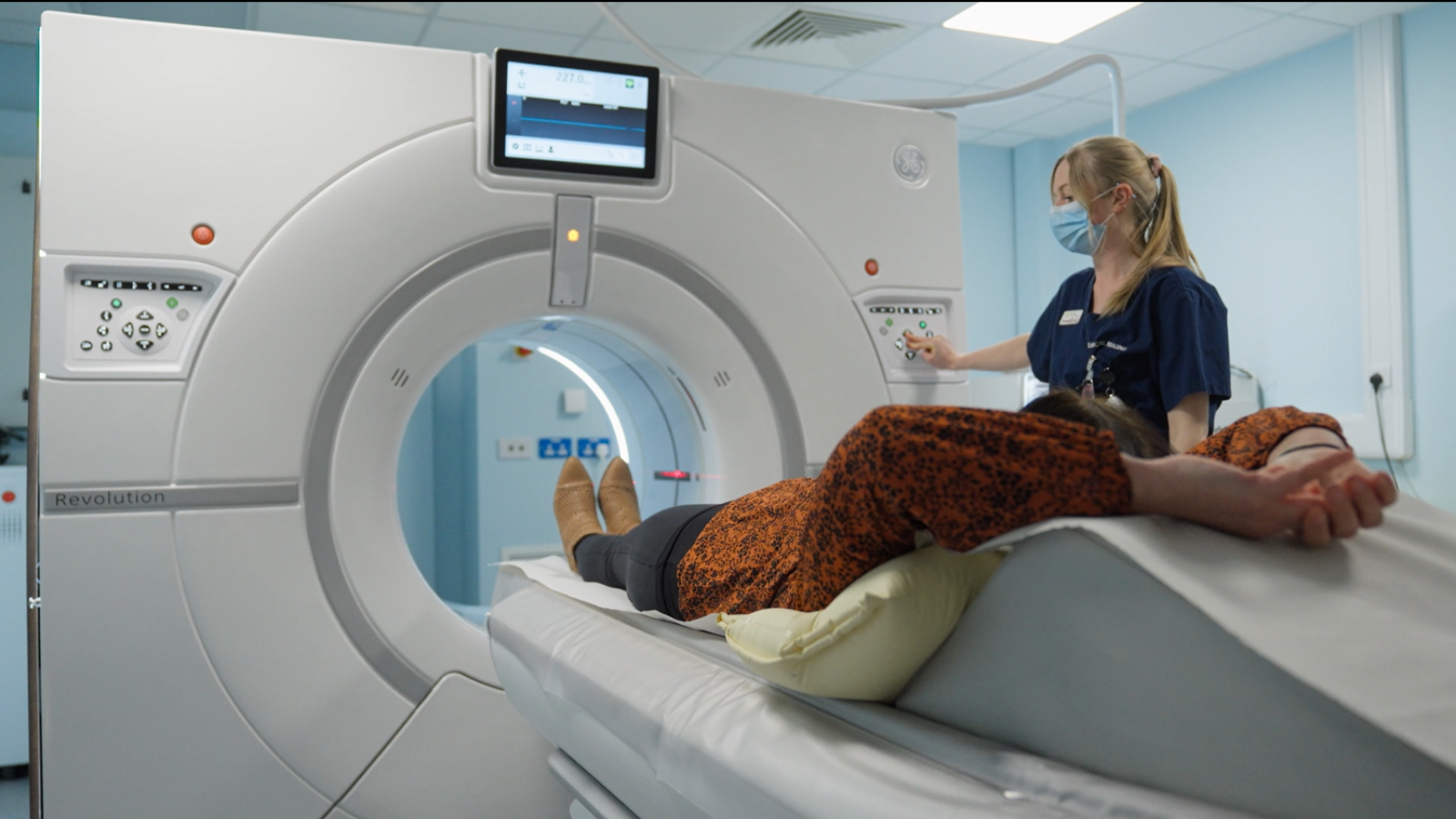
Unlocking the future of lung cancer screening: How DART is redefining early diagnosis
October 23, 2023
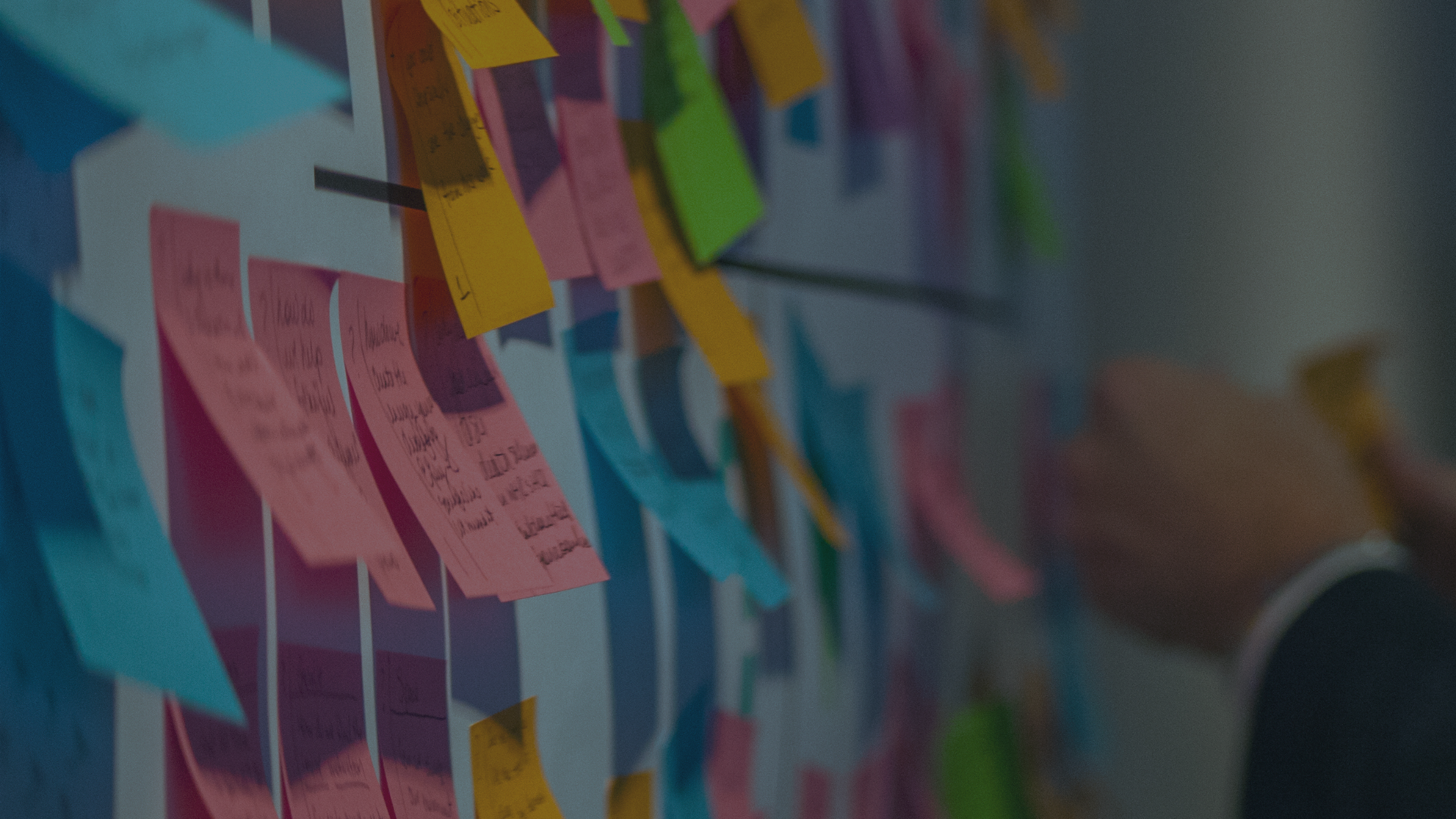
Comparing imaging techniques to diagnose lung cancer – looking for advisors
May 25, 2023
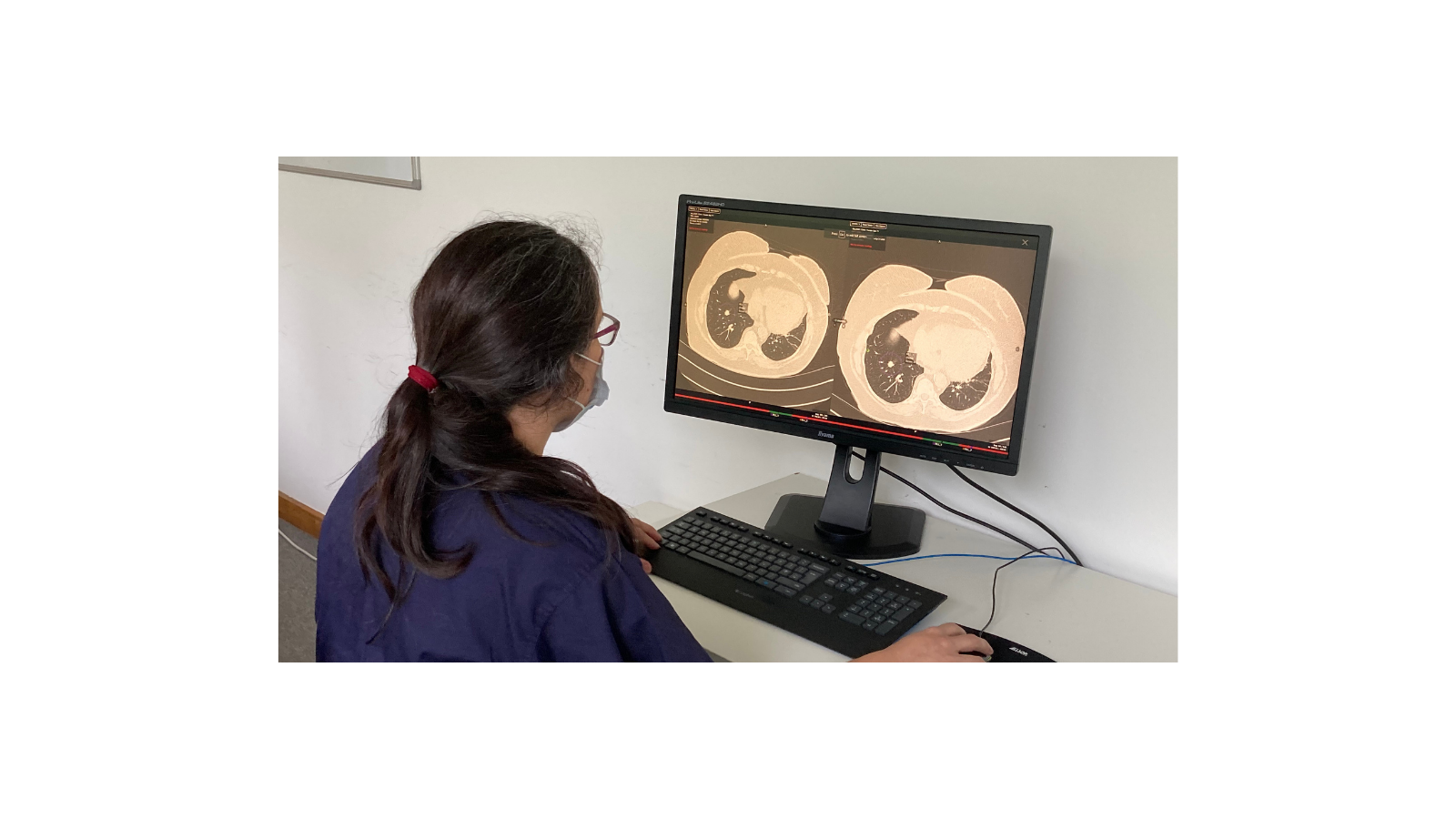
CanPredict: Lung cancer risk prediction
April 13, 2023