Automated Annotator: Capturing Expert Knowledge
October 27, 2021
A paper on “Automated Annotator: Capturing Expert Knowledge for Free” by the DART digital pathology and radiomics team was presented at EMBC, the 43rd Annual International Conference of the IEEE Engineering in Medicine and Biology Society Intervention, November 1-5, 2021. EMBC is the flagship conference of the IEEE EMB Society and the peer-reviewed conference proceedings attract submission of innovative work through the international gathering.
Paper Abstract: Deep learning enabled medical image analysis is heavily reliant on expert annotations which is costly. We present a simple yet effective automated annotation pipeline that uses autoencoder based heatmaps to exploit high level information that can be extracted from a histology viewer in an unobtrusive fashion. By predicting heatmaps on unseen images the model effectively acts like a robot annotator. The method is demonstrated in the context of coeliac disease histology images in this initial work, but the approach is task agnostic and may be used for other medical image annotation applications. The results are evaluated by a pathologist and also empirically using a deep network for coeliac disease classification. Initial results using this simple but effective approach are encouraging and merit further investigation, especially considering the possibility of scaling this up to a large number of users.
Co-author, Tapabrata (Rohan) Chakraborty said “We present a pilot demonstration to show how AI maybe be used to learn the annotations of pathologists from a relatively few examples without interrupting their workflow, and then use that knowledge to annotate new images in an automated fashion, thus reducing time and cost. This paper is based on an earlier work on coeliac disease histology data by current DART members and past students, and it is being exploited and extended as a generalised approach in the current DART project on lung histology data.”
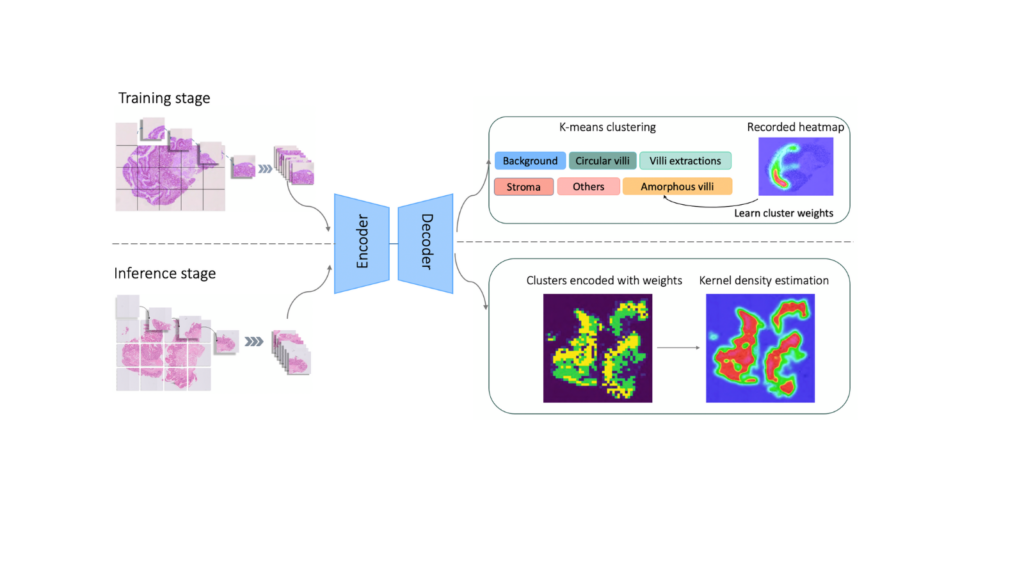
Important: DART Programme Data Email Address Update
March 17, 2025
Thank you to all the sites which are sending data to the DART Programme. Please note that our email address…
Update on the NHS Lung Cancer Screening Programme
March 4, 2025
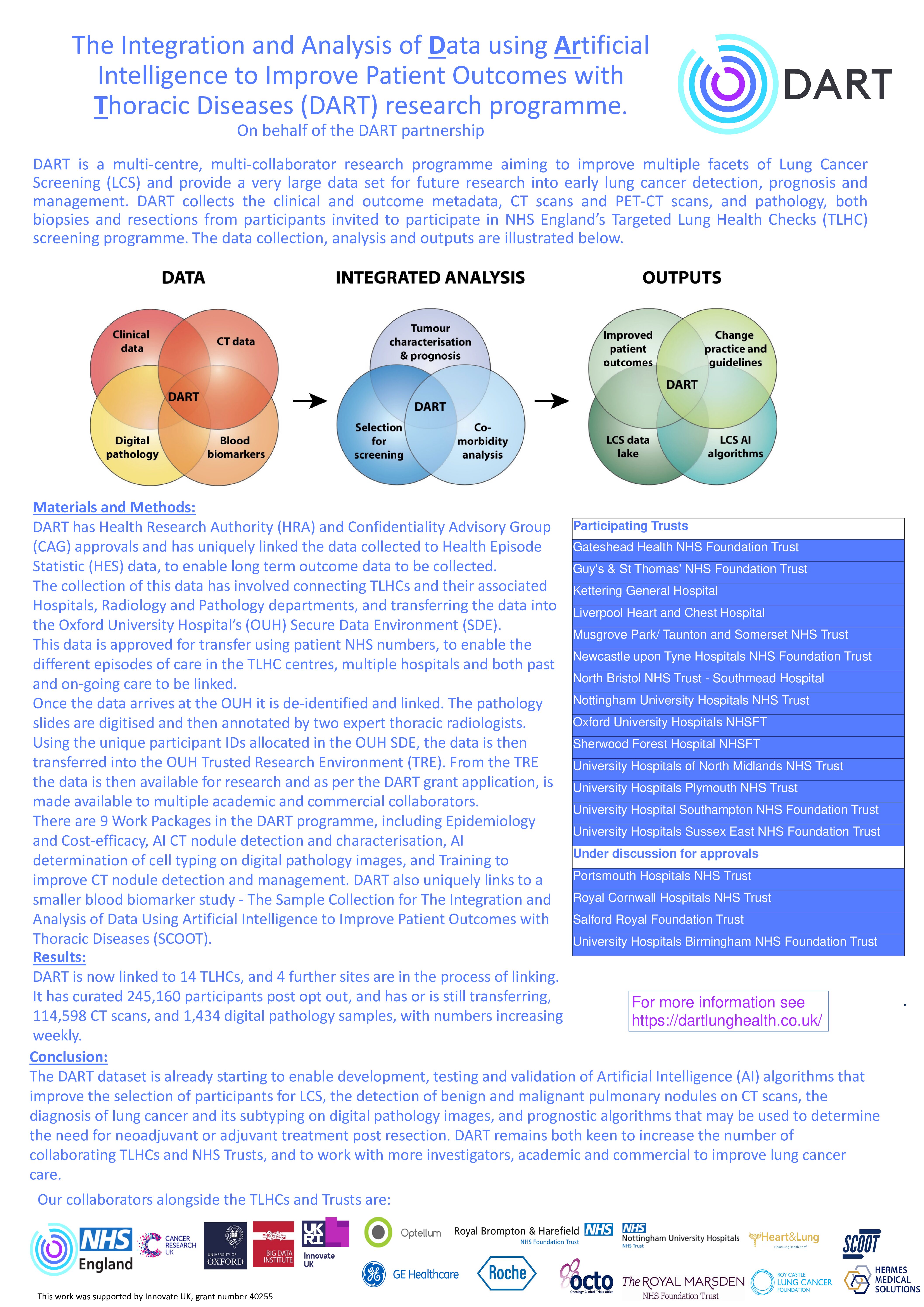
DART at OxCODE
January 29, 2025
Active data enrichment by learning what to annotate in digital pathology
March 11, 2024